What is AI Self Evaluation: An Overview of Assessing and Improving AI Responses
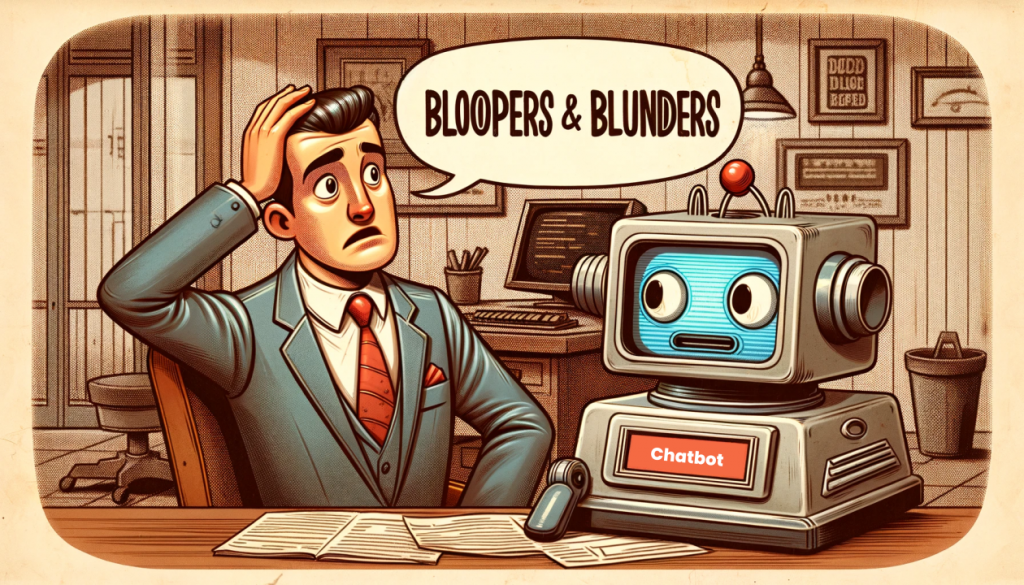
This blog has been adapted from our weekly newsletter. For more updates click here.
Generative AI is the new electricity. It is changing the way the world functions at large. Just like electrification majorly brought about the Second Industrial Revolution and then just became a very natural part of our day-to-day existence, Generative AI is on the same path.
However, despite all the amazing use cases, when it comes to providing great customer-facing solutions, Generative AI isn’t the answer for enterprises. According to a report by BCG of 2,000 global executives, more than 50% still discourage GenAI adoption. Problems of hallucination, limited traceability, and compromised data privacy are just some of the major concerns they have.
There have been multiple instances of AI hallucinations, some of which were actually hilarious. Douglas Hofstadter, the Pulitzer Prize-winning author of Gödel, Escher, Bach, got some brilliant answers from ChatGPT.
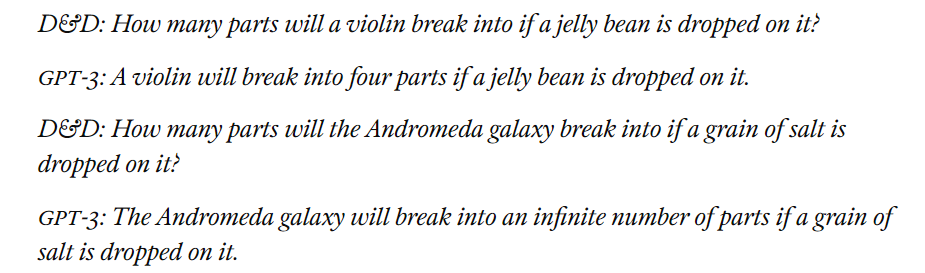
These concerns render GenAI unreliable, especially for customer-facing solutions.
Make every answer count with high relevance
As we discussed in our previous edition, RAG (Retrieval Augmented Generation) is an architectural approach that can improve the efficacy of LLMs. Conversational AI relies on two key elements: Intent Detection and OpenAI-powered Generative Q&A.
Intent detection, as the name suggests, understands customers’ underlying needs behind each question, providing accurate solutions. Generative Q&A draws from internal sources like websites, PDFs, and Slack to provide business-specific answers.
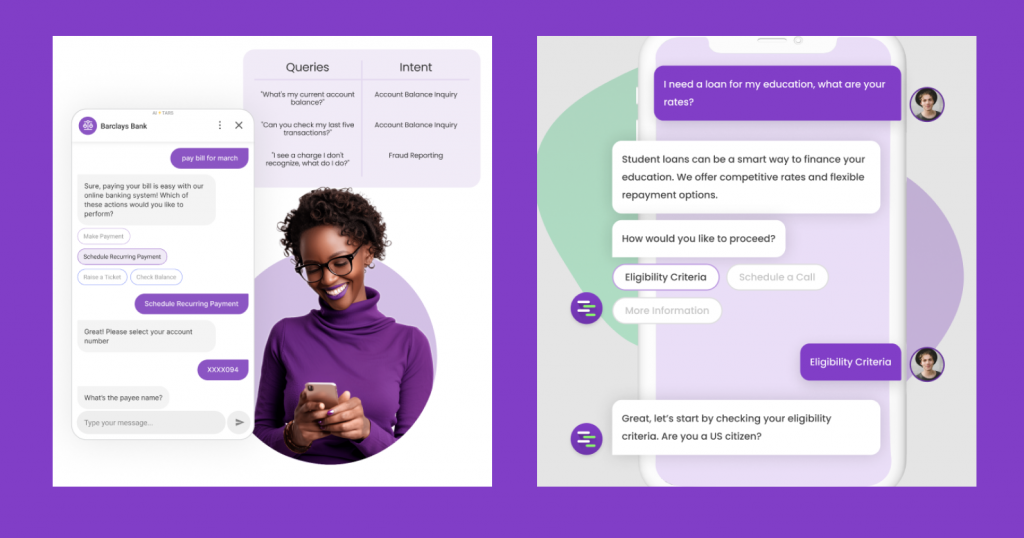
The RAG-based LLM app breaks down information into smaller bits, and the restricted boundary around the knowledge base reduces hallucinations and bias.
However, it is necessary to manually test the responses to hundreds of questions. It can take hours of your time. So, what’s the solution?
To help you save many hours and make Conversational AI accurate and reliable, we have worked on Evaluation AI.
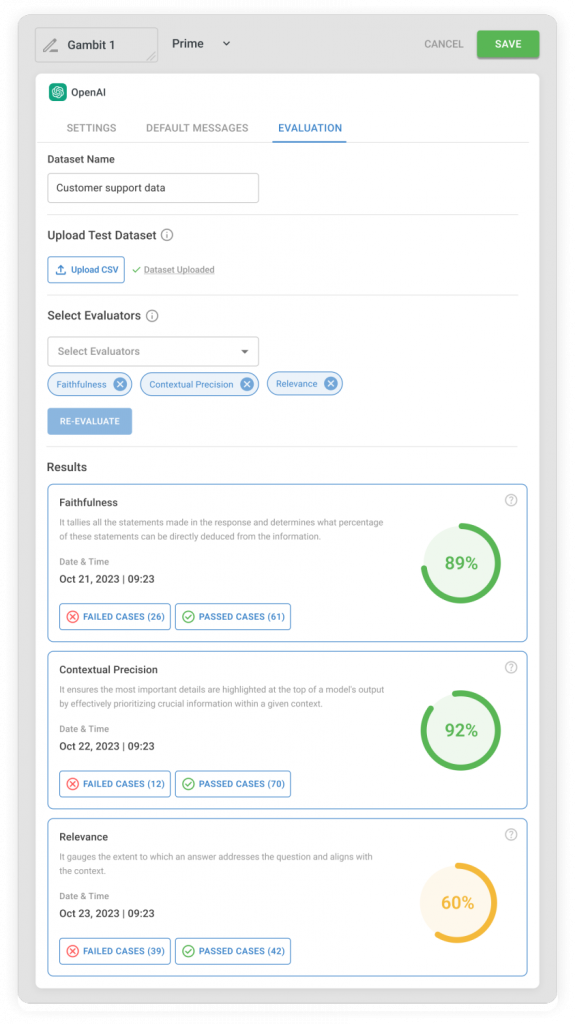
It operates on four key parameters aimed at optimizing response relevance and accuracy. Let’s gain a better understanding of them.
Four parameters for AI self-evaluation
General Evaluation
Answer Relevancy: As the name suggests, the parameter evaluates the pertinence of a given response to the provided prompt. It gauges the extent to which an answer addresses the question and aligns with the context provided. A lower score points out a lot of irrelevant information in the output that can often throw off or overwhelm the end user. This evaluation is especially crucial in the case of Intent Detection.
Faithfulness: With faithfulness, essentially, we’re testing how closely the response aligns with the information provided in the context. It tallies all the statements made in the response and determines what percentage of these statements can be directly supported or deduced from the background documents or information. This metric doesn’t rely on a predefined truth; it’s solely based on the coherence between the response and its contextual foundation.
Retrieval Evaluation
Contextual Precision: Context Precision serves as a metric for evaluating the effectiveness of natural language processing models. It measures the extent to which all relevant information within a given context is accurately prioritized in the model’s output. In simple words, the details that matter the most are prioritized, and the goal is for all pertinent details to be ranked at the top, ensuring optimal relevance and accuracy.
Contextual Recall: Contextual recall measures the extent to which the retrieved context matches the pre-defined answer, treated as the ground truth. In an ideal situation, all components of a standard response should be present in the output. A higher score indicates better alignment between the retrieved context and the actual answer.
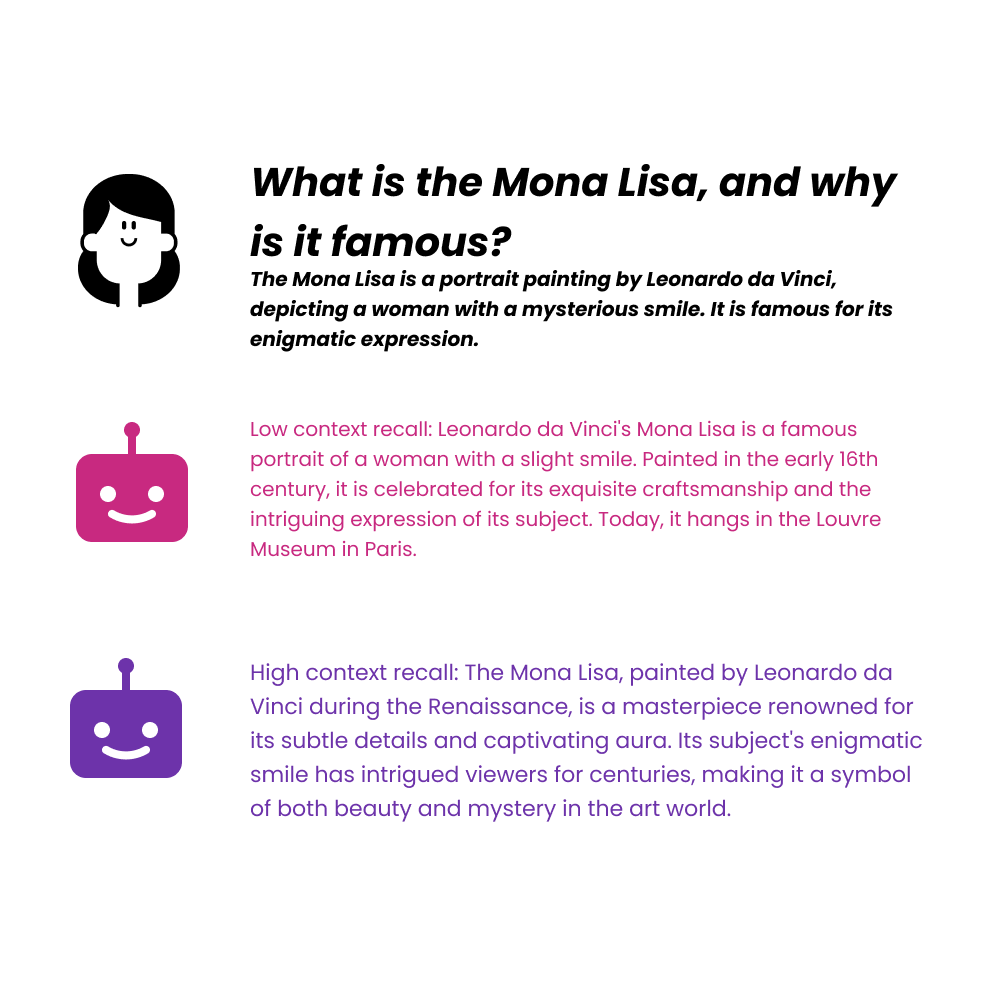
We aim to make Conversational AI a refined solution for complex problems and a trustworthy AI Agent with AI Self Evaluation.
Learn why businesses trust us to automate their pre-sales and post-sales customer journeys.
Recommended Reading: Check Out Our Favorite Blog Posts!
Recommended Reading: Check Out Our Favorite Blog Posts!
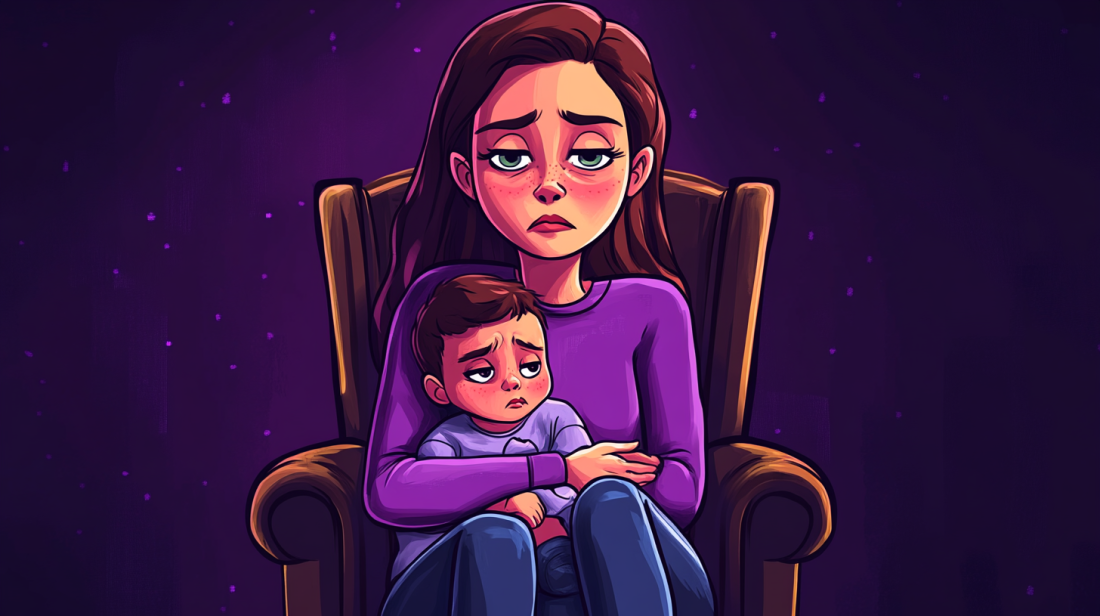
AI Agent builders, we need to have a chat about AI’s gender bias
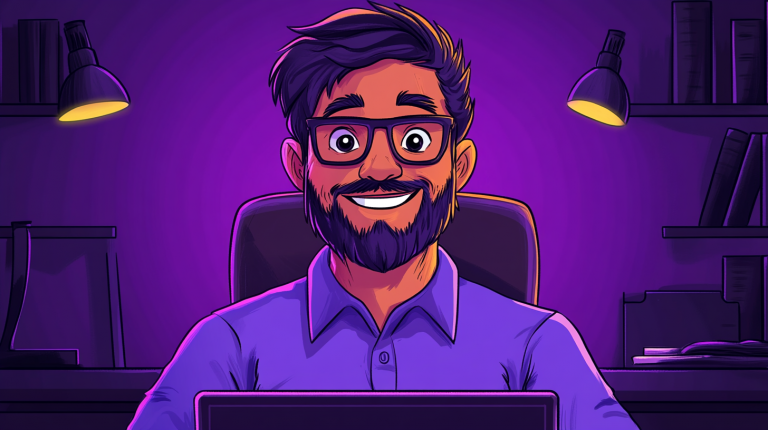
Time is money, so we created AI Agents that help founders save both!
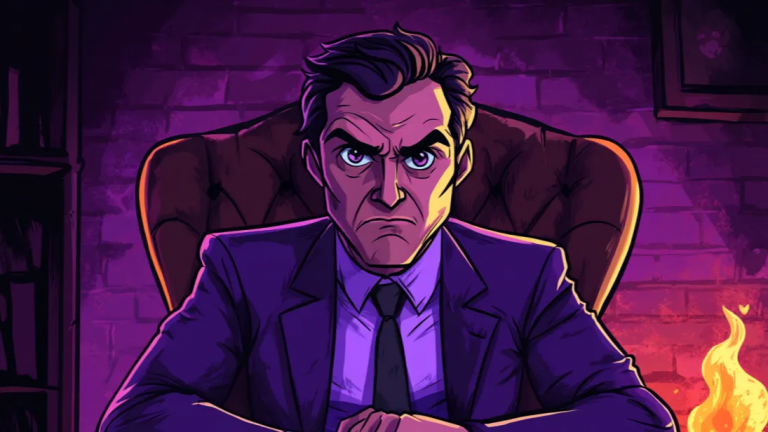
How to Get Your Startup Idea Validated by Paul Graham or Kevin O’Leary? AI Agent to the Rescue
Our achievements in numbers
7+
years in the Enterprise Conversational AI space
700+
global brands that we’ve worked with
50m+
customer conversations automated
10+
countries with deployed Chatbots