AI Self Evaluation in Banking: Navigating Risks and Ensuring Reliability
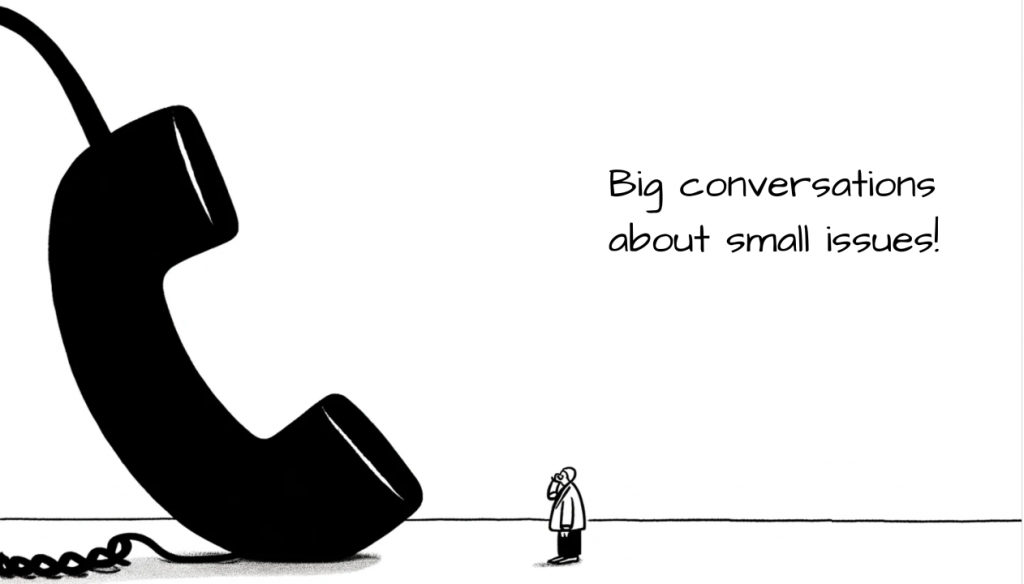
This blog has been adapted from our weekly newsletter. For more updates click here.
The first ever chatbot dates back to 1966, when an MIT professor named Joseph Weizenbaum created Eliza. However, it is only in the past decade that Conversational AI has become mainstream.
In the realm of AI-powered banking solutions, the revolution truly took off in the mid-2010s, and today we are on the brink of a Conversational AI evolution. In 2022, nearly 37% of the U.S. population is estimated to have interacted with a bank’s chatbot. This number is only expected to grow drastically each year.
However, as with most disruptive technologies, the evolution of chatbots has brought along new challenges as well. Especially when we talk about the use of Conversational AI in the financial industry, the problems extend beyond bias and hallucination. They are a little more complex than that.
Can banks truly rely on AI for better Customer Experience?
“What is worse is that there is no way to contact a person who can actually resolve the situation.”
AI operates based on the datasets it is trained on, which can introduce a degree of inflexibility when dealing with complex questions. In cases like consumer complaints or dispute settlement, many individuals experience significant negative impacts due to the technical limitations of chatbots. These issues include wasted time, frustration, receiving incorrect information, and incurring additional fees. The problems are particularly severe when customers cannot obtain personalized support.
This issue is further complicated for customers with limited English proficiency when using chatbots for resolutions.
For example, this customer complaint illustrates the point:
“Virtual assistant kept sending me in circles.”
Artificial intelligence lacks the sense of empathy that a human possesses when handling problems requiring more sensitivity. Automated responses often follow rigid scripts or refer customers to extensive policy documents or FAQs, which might leave them helpless. Chatbots can sometimes fail to address a customer’s issue, trapping them in endless questions and answers without resolution. If a customer’s problem exceeds the chatbot’s capabilities, it can become stuck.
For instance, a customer complained:
“It hallucinates.”
Hallucinations and inaccuracies are major concerns with AI implementation. AI-generated hallucinations can lead to false narratives and misleading information, particularly in financial advice on investments and loans. This can result in incorrect plans or details, damaging the bank’s reputation, and potentially leading to lawsuits. Hallucinations can arise from various factors, including model complexity and training data inaccuracy, but the outcome is always a misinformed or agitated customer.
“Is the chatbot compliant?”
With open APIs, some people may believe anyone can build a decent chatbot. However, that’s not the case. Automated solutions backed by unreliable technology pose a major threat to data privacy and are more prone to hallucinations. Also, inferior products can often struggle with the complex compliance needs of financial institutions. With many options available on the market, distinguishing the good from the bad can be challenging.
“Is my data safe?”
To offer personalized solutions, chatbots need access to personal and sensitive customer information. This raises significant concerns about data privacy, compliance, and customer security. A minor slip-up with customer information can lead to financial fraud or misuse of personal data, making it more vulnerable to third-party service providers involved.
How do we aim to tackle these problems: AI Self Evaluation in Banking
Our aim at Tars is to understand each concern surrounding Conversational AI and build realistic solutions that not only meet compliance standards but are reliable, accessible, and secure.
AI Experience
Choosing inferior solutions can save money in the short term but can cost more in the long run due to noncompliance and unreliability. At Tars, we have been building AI Agents for 8 years. We have identified and addressed many blind spots and bottlenecks while working with clients such as Angel One, American Express, VM Group, Indiana INBiz, and Bajaj Finserv, giving us a deep understanding of the financial landscape.
Preventing Doom Loops
Humans excel at handling complex or unforeseen problems with empathy. When a customer conversation runs in circles without resolution, our system detects and provides the option to talk to a Live Agent. This prevents the customer from entering endless loops, allowing an agent to step in and provide a prompt resolution.
AI Evaluation
Our AI Self Evaluation system further enhances the relevance of each answer by judging responses based on four parameters:
- Answer Relevancy: Gauges how well an answer addresses the question and aligns with the context.
- Faithfulness: Tallies the statements in the response to determine the percentage that can be directly deduced from the information.
- Contextual Precision: Ensures crucial details are prioritized at the top of the model’s output.
- Contextual Recall: Measures how well the retrieved context aligns with the correct response.
AI Accuracy
Breaking information into smaller parts and setting boundaries around the knowledge base improves the utility of LLMs with specific data sources. The RAG-based LLM application combines retrieval and generative models.
- Chunking: This means breaking down input text into smaller, meaningful segments. It helps the retrieval system accurately identify relevant passages for generating responses.
- Vector Databases: These manage data stored as vectors. They capture semantic similarities between words and phrases, enabling contextual relevance in responses.
- Embedding Model: It converts the user’s query and retrieved documents into dense vectors that capture their meaning, making it easier to find the most relevant information.
Using this approach, the system can fetch the most accurate source documents by comparing the similarity of their embeddings to the query. These documents, along with the user query, provide context for the LLM to produce a precise answer.
Structured Menu Fallback
If a customer can’t easily describe a complex problem, our UX switches to a simple fallback menu. This helps them find and select specific issues quickly, making the experience smoother and more effective.
Integration and Compliance
We take data privacy and security very seriously, offering multiple integration options to ensure customer information transfers directly to your CRM, staying between you and the customer. No third party can access the information. Additionally, we comply with SOC 2, GDPR, ISO, and HIPAA standards.
Learn why businesses trust us to automate their pre-sales and post-sales customer journeys.
Recommended Reading: Check Out Our Favorite Blog Posts!
Recommended Reading: Check Out Our Favorite Blog Posts!
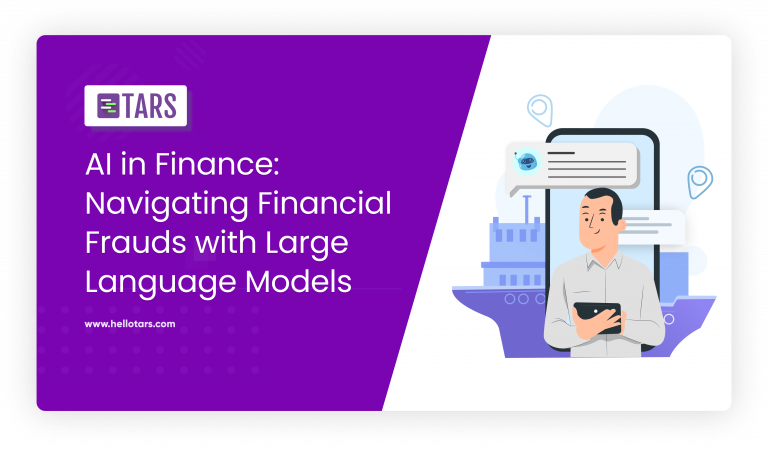
AI in Finance: Navigating Financial Frauds with Large Language Models
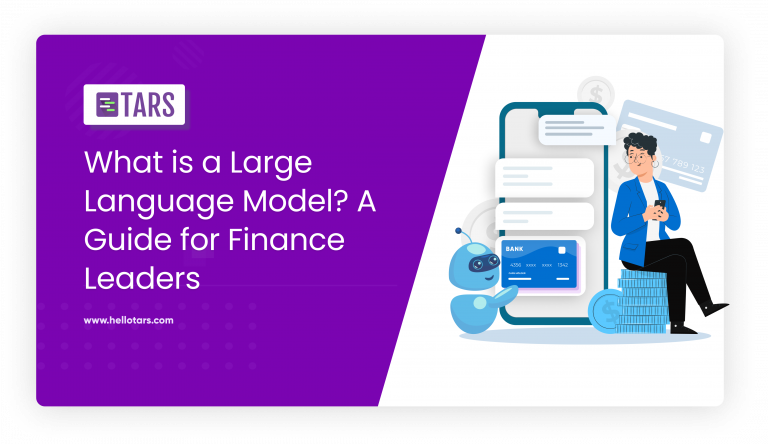
What is a Large Language Model? A Guide for Finance Leaders
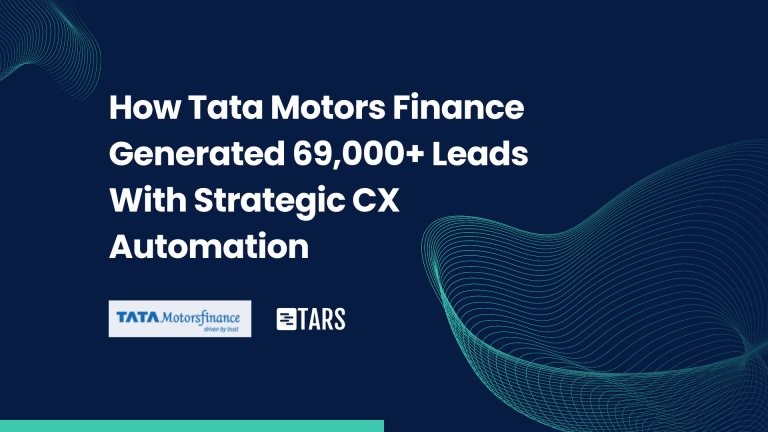
How Tata Motors Finance Generated 69,000+ Leads With Strategic CX Automation
Our achievements in numbers
7+
years in the Enterprise Conversational AI space
700+
global brands that we’ve worked with
50m+
customer conversations automated
10+
countries with deployed Chatbots