AI in Finance: Navigating Financial Frauds with Large Language Models
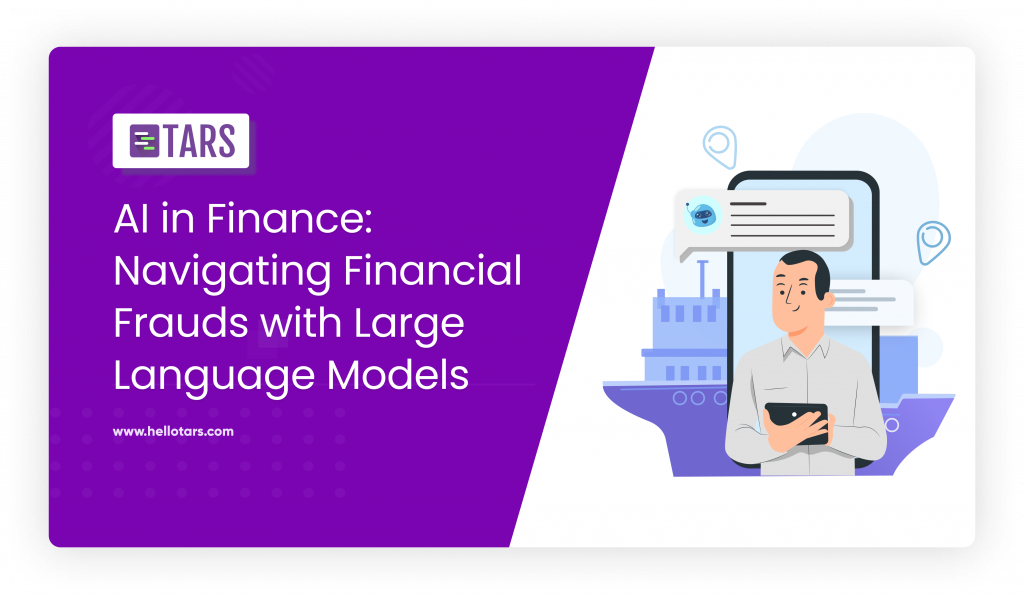
Imagine you have two tools in your toolkit. One is an old, trusty magnifying glass that’s been passed down through generations. It’s seen its fair share of mysteries and has helped solve many. The other is a brand-new, high-tech radar system, capable of scanning vast areas in seconds. The magnifying glass represents our traditional ML models, and the radar? That’s our Large Language Models (LLMs).
Traditional ML Models: The Trusty Magnifying Glass
These models have been around for a while:
- Detailed Inspection: They’re great at zooming in on specific problems. They’ve been trained to spot the usual suspects, especially in areas like credit card transactions or insurance claims.
- Structured Approach: They love working with organized data. Give them a neat list or a well-arranged table, and they’ll sift through, looking for any signs of mischief.
- Known Boundaries: But as the world of finance grew and evolved, with new methods of transactions and a plethora of online platforms, these models sometimes struggled to keep up.
LLMs: The All-Seeing Radar
LLMs are the new kids on the block:
- Broad Surveillance: They don’t just focus on known problems. They’re always on the lookout, catching new and evolving tricks, from deceptive loan applications to unusual online shopping patterns.
- Deep Understanding: They aren’t limited to just numbers and tables. They delve into feedback, customer chats, reviews, and more, trying to understand the context and the sentiment.
- Adaptive Learning: Their strength lies in their ability to learn and adapt. As they process more information, they get better, making them invaluable in today’s dynamic financial landscape.
Addressing a Spectrum of Financial Challenges
- Loans: People sometimes aren’t entirely honest on loan applications. They might exaggerate their income or downplay their debts. While older models might catch blatant inconsistencies, LLMs can delve deeper, analyzing patterns and inconsistencies that might not be immediately obvious.
- Insurance: There are instances where individuals might try to game the system, exaggerating damages or even orchestrating accidents. LLMs can meticulously go through claim details, spotting anomalies or patterns that hint at deception.
- Online Shopping: As more and more people turn to online platforms for their shopping needs, the risk of fraudulent transactions increases. While traditional models might flag large, suspicious purchases, LLMs can analyze buying habits, review patterns, and even the language used in feedback to detect potential fraud.
- Banking: Unusual money transfers, especially frequent small amounts, can be a red flag. LLMs keep a vigilant eye on these patterns, ensuring banks are alerted to any suspicious activity.
- Investments: The world of investments isn’t immune to deception. Some might spread false information or rumors to manipulate stock prices. LLMs can scan through vast amounts of data from chats, forums, and news articles, identifying and flagging potential misinformation.
- Credit Cards: With the convenience of swipe-and-go, credit card frauds have become more common. LLMs can monitor transaction patterns, locations, and even times to spot any out-of-the-ordinary activity.
Combining the Best of Both Worlds for a Secure Financial Future
By harnessing the strengths of both traditional models and LLMs, we’re setting ourselves up for a safer financial environment. While the traditional models lay a solid foundation with their tried-and-tested methods, LLMs bring in a fresh perspective, equipped to handle the complexities of today’s financial world.
Coming Up:
This is part 2 of our LLM in Finance series. Stay updated for our upcoming posts on the role of LLMs in finance. Stay tuned!
LLM Amplifying Customer Support:
Imagine customer support where repetitive tasks are automated by LLMs, freeing humans to tackle complex issues and provide a personal touch. We’re not just speeding up responses but elevating the human role to focus on deeper customer relations. Welcome to efficient yet meaningful customer interactions 🧡
Dive in for a free automation consultation with us 🚀. Witness firsthand how we channel the might of LLMs to revolutionise customer support automation.
Hello! I'm a problem solver at Tars, passionate about blending AI, design, and automation in the SaaS realm. With roles like Design Lead, Engineering Lead, and Writer at Hacker Noon under my belt, I've nurtured a mix of skills that drive innovation. At Tars, I help companies automate customer support in both public sector and private enterprises, making processes smoother. My goal? To create solutions that not only answer today's issues but anticipate tomorrow's questions. I share my insights on Large Language Models, Transformers and other emerging technologies at Hacker Noon, I also help startups communicate their visions clearly. I've learned that understanding users, showing empathy, and asking the right questions are the stepping stones to success. I'm always open to connecting with professionals who share my excitement for technology and its power to change lives. If you're curious about AI, design, or automation, feel free to reach out. Let's create something impactful together!
Learn why businesses trust us to automate their pre-sales and post-sales customer journeys.
Recommended Reading: Check Out Our Favorite Blog Posts!
Recommended Reading: Check Out Our Favorite Blog Posts!
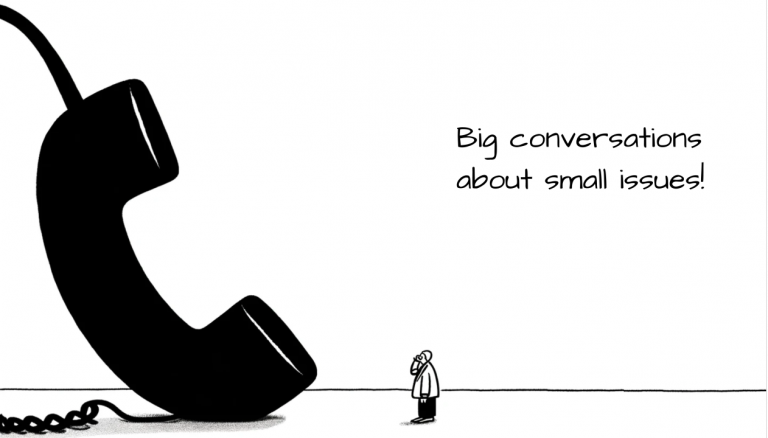
AI Self Evaluation in Banking: Navigating Risks and Ensuring Reliability
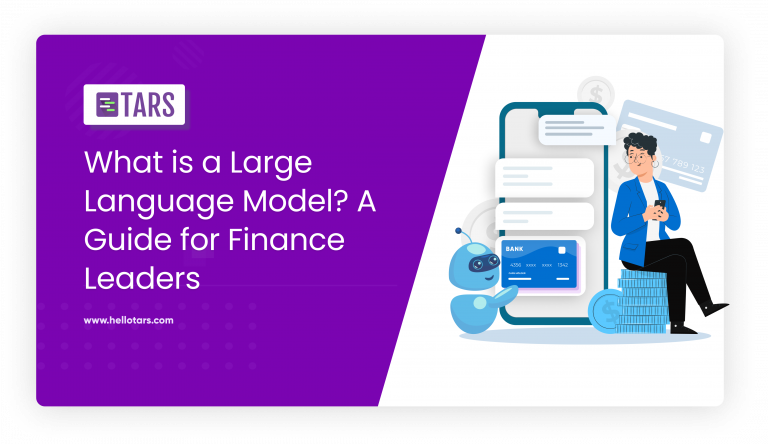
What is a Large Language Model? A Guide for Finance Leaders
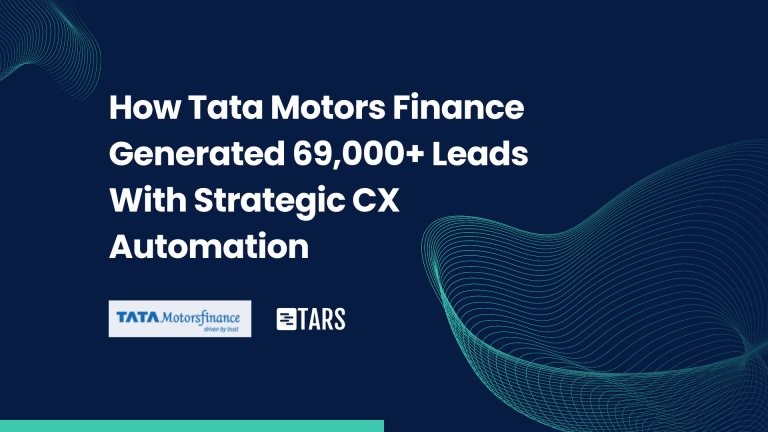
How Tata Motors Finance Generated 69,000+ Leads With Strategic CX Automation
Our achievements in numbers
7+
years in the Enterprise Conversational AI space
700+
global brands that we’ve worked with
50m+
customer conversations automated
10+
countries with deployed Chatbots